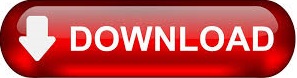

The new archaeological discoveries included eight important line segments with a 9:6 proportional relationship (which represents the balance of Yin and Yang) and two important line segments with a 9:5 proportional relationship (which represents the supreme imperial power) in the capitals of the Western Han dynasty as well as the Sui and Tang dynasties, and 16 contraposition lines in a positive direction or oblique 45° direction (which reflects the close relationship between urban elements and natural elements). The research results indicated that this analysis method was feasible, effective, and easy to promote. The drawings were exported after screening, and a visual expression was realized with Illustrator software. The decision model was used to test the geographic information base, and the information of strong correlations between urban objects and natural objects was outputted. Then, Python was used to construct a “decision model of spatial relationship between urban elements and natural elements”, and rules as well as parameters were set through man–machine collaboration. Firstly, satellite images, elevation maps, urban ichnographies, and literature materials were collected and sorted to deeply understand the cultural thoughts involved in ancient urban planning based on this, key element points were marked and rechecked on the spot, and the above drawings were accurately superimposed by GIS technology to form a geographic information base that integrated multisource information. This paper takes them as its subjects and puts forward a method to study the spatial relationship between ancient cities and the natural environment by combining spatial information technology and cultural analysis. The capitals of the Western Han dynasty as well as the Sui and Tang dynasties were some of the greatest cities in the world at the time.
#G239 inspirit program how to#
How to combine science and technology with the humanities in the research on ancient cities to reveal ancient peoples’ urban planning thoughts is worthy of in-depth study. EHEM seems most useful to optimize promising strategies, yet EM methods are complex and might only add value when stakeholders are willing to consider multiple solutions to a problem and adopt flexible research and adoption strategies. EM seems most suited in the very early phases of innovation to explore existing uncertainty and many potential strategies. EM showed that a policy with a population in which most patients had severe intracerebral hemorrhage was most robust, regardless of MIS effectiveness, complications, and costs.ĮHEM and EM were found to complement each other. EHEM provided cost-effectiveness thresholds for MIS effectiveness, assuming fixed MIS costs. EM aims to find robust strategies, which give relatively good outcomes over a wide range of plausible futures. Where in EHEM the focus is on the value of technology, while accounting for the uncertainty, EM focuses on the uncertainty. We applied EM and EHEM to an early evaluation of minimally invasive endoscopy-guided surgery (MIS) for acute intracerebral hemorrhage and compared these models to derive differences, merits, and drawbacks of EM.ĮHEM and EM differ fundamentally in how uncertainty is handled. Our aim was to evaluate the use of EM for early evaluation of health technologies. The field of decision making under deep uncertainty uses exploratory modeling (EM) in situations when the system model, input probabilities/distributions, and consequences are unknown or debated. In early stages, the consequences of innovations are often unknown or deeply uncertain, which complicates early health economic modeling (EHEM). The Exploratory Modeling Workbench enables users to easily perform exploratory modeling with existing models, identify the policy-relevant uncertainties, assess the efficacy of policy options, and iteratively improve candidate strategies.
#G239 inspirit program series#
It is designed to (i) support the generation and execution of series of computational experiments and (ii) support the visualization and analysis of the results from the computational experiments. The Exploratory Modeling Workbench is implemented in Python. This paper presents an open source library supporting this. This is achieved by conducting series of computational experiments that cover how the various uncertainties might resolve. Exploratory modeling aims at exploring the implications for decision making of the various presently irresolvable uncertainties. A key idea shared among these is the use of models for exploratory rather than predictive purposes.

There is a growing interest in model-based decision support under deep uncertainty, reflected in a variety of approaches being put forward in the literature.
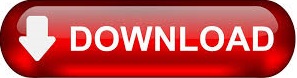